Intelligent transportation systems (ITS) have the potential to transform road transportation and personal mobility. Advances in information and communication technologies are leading to the emergence of intelligent vehicles (IVs) that involve many challenges from vehicle design to control, coordination, decision-making and human interactions. Using a combination of Engineering and Statistics, scientists of the EU-funded BITS project will work on a novel methodology for uncertainty identification and efficient estimation of various unknown parameters of ITS. They will conduct physical tests to validate the proposed methodologies. This work will provide an accurate representation of a network of IVs and will contribute towards the deployment of IVs in real traffic networks.
Road Transportation Systems account for (in EU alone):
- 25% of total energy consumption
- 75% of fuel consumption
- 18% of total GHG emissions
- 30,000 fatalities per year
- Traffic congestion accounts for one work week per year per person and 2% of EU’s GDP
Solution: Increase existing infrastructure capacity with the use of Intelligent Transportation Systems (ITS).
The future of transportation systems is even more exciting as Intelligent Vehicles (IVs) are expected to revolutionize transport.
Benefits:
- Significantly reduce the number of accidents
- Minimise environmental effects
- Enhance mobility of elderly, disabled people
- Reduce traffic congestion
- Reduce number of parking spaces
The deployment of IVs requires many advances from vehicle design to control, coordination, decision-making and human interactions.
Challenges:
- In order to evaluate and minimize risks in their dynamic environment, it is necessary to have accurate mathematical models of the situation.
- The uncertainty associated with the models has to be estimated in order to take proper decisions and regulate driving behaviour accordingly.
The Bayesian approach is a method of statistical inference, a proven and mathematically rigorous framework to update any prior beliefs about an uncertain parameter or model, as more information becomes available and relies on Bayes’ theorem.
- Posterior distributions are derived for the unknown parameters and quantities of interest, which are the required representation of uncertainty, and are used to make predictions of the system through the posterior predictive distributions.
- Bayesian nonparametric methods are able to modify the model’s complexity based on the complexity of the data.
The Bayesian computation tools will allow us to tackle enormously complex problems such as the dynamical problems arising from the study of IVs due to lack of knowledge about the environment or parameters.
The aim of this project is to transfer Bayesian statistical methodologies in the area of Intelligent Transportation Systems (ITS) and contribute towards the efficient decision-making and fault diagnosis capabilities of intelligent vehicles (IVs), as well as improve their collective behavior when interacting with other vehicles and the road infrastructure.
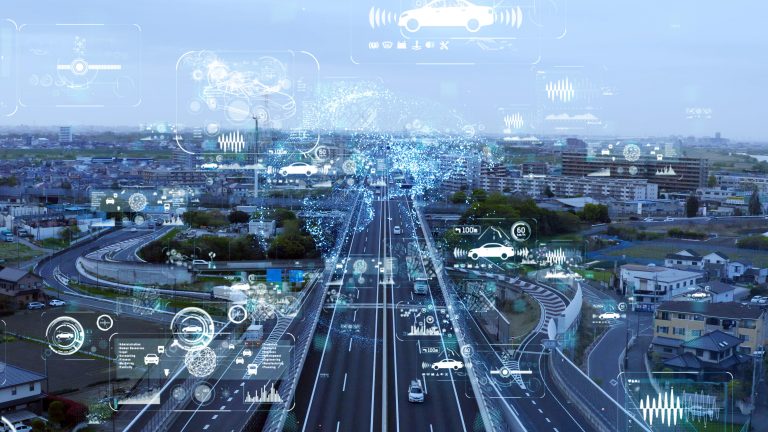
This project will provide an accurate representation of a network of IVs along with the probabilistic uncertainty concerning the vehicles, to gain insights on their performance, reliability and safety and improve the predictability of the system. As part of the project, physical tests will be conducted to validate the proposed methodologies and with the use of computer science tools will evaluate and minimise risks in the dynamic environment of IVs.
BITS is highly interdisciplinary and combines methods from Engineering, Statistics and Computer Science. It will bring together the best practices and state-of-the-art methods of different fields and support the Fellow, who has a strong background in Bayesian learning, to make significant contributions via collaboration with the supervisors, who are experts in ITS, and with leading groups in the field.
The project will contribute towards the deployment of IVs in real traffic networks and our everyday lives.
“This project brings together the best of two worlds, Intelligent Transportation Systems and Bayesian Statistics aiming to develop novel methodologies for the deployment of Intelligent vehicles in our everyday lives.”