Accurately estimating wildfire propagation is crucial for effective firefighting strategies and informed decision-making. This research introduces a novel solution that utilizes an autonomous UAV agent equipped with advanced sensing capabilities, alongside a wildfire spread model and a state-estimation methodology based on the Ensemble Kalman Filter (EnKF). By strategically directing the UAV to critical areas ahead of the fire, it gathers real-time data on fuel types and weather conditions. This data is combined with historical information to enhance the accuracy of wildfire propagation predictions. The updated data then informs both the wildfire spread model and EnKF, improving estimations of wildfire behavior and subsequently refining UAV path planning. The effectiveness of this approach is demonstrated in a simulated environment, showcasing its potential to enhance both the estimation of wildfire propagation and the accuracy of current fire perimeter assessments.
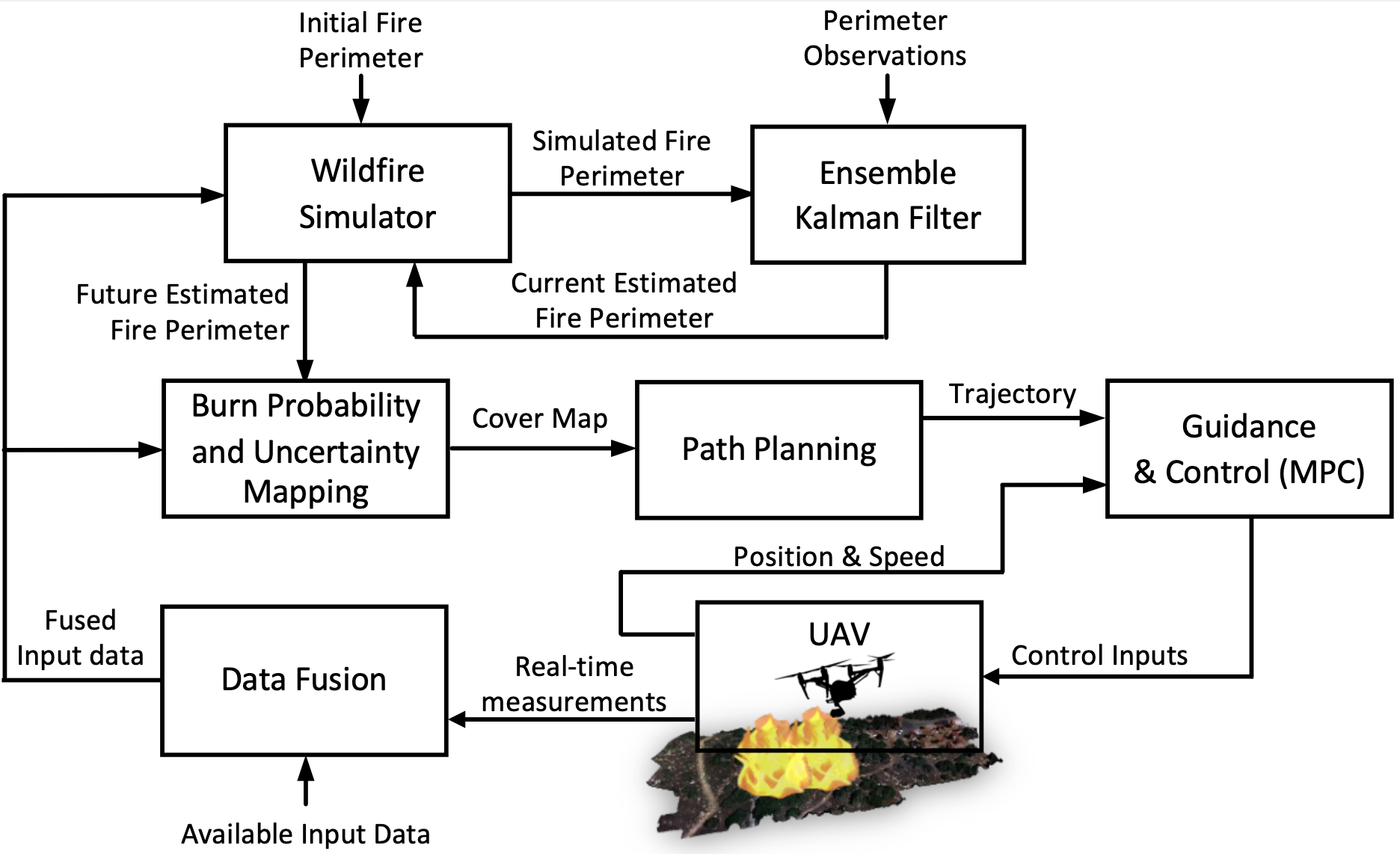
Key contributions of this work include:
a) Development of a wildfire simulator and EnKF that utilize continuously updated input data to provide estimations of the current fire perimeter and predict future fire spread over a specified horizon, accounting for input data uncertainties.
b) A strategy for guiding the UAV to the most data-deficient regions ahead of the fire. This involves generating a landscape uncertainty map by calculating burn probability based on future fire perimeter predictions and integrating data uncertainty values.
The details of the proposed approach can be found in the references listed below.
References: